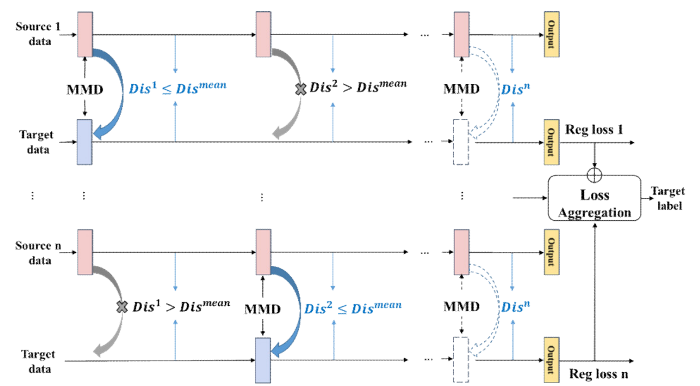
Industrial Time Series Prediction Framework
In view of the problems of insufficient data and algorithm cold start in the modeling process of complex industries such as metallurgy, big data research group under Digital Factory Department of Shenyang Institute of Automation (SIA), the Chinese Academy of Sciences (CAS) has proposed a prediction method of industrial process time series based on dynamic Transfer learning under limited data volume. This method aims at improving multi-step time series prediction accuracy and reducing calculation costs.
Industrial time series, as a kind of data that responds to production process information, can be analyzed and predicted for effective monitoring of industrial production processes. However, data shortage and algorithm cold start in industrial modeling process can be caused by complex working conditions, change of data acquisition environment and short running time of equipment, hindering the accuracy of the existing data-driven industrial time series prediction algorithm.
To address is issue, the research team proposed a new time series prediction method for industrial processes under limited data based on dynamic transfer learning. Historical data are divided into multiple batches, and then a new multi-source transfer learning framework with dynamic maximum mean difference (MMD) loss is established according to the distribution distance between each batch of historical data and the limited target data at the current moment. The framework also combines multi-task learning methods to establish multi-step prediction model for online learning in industrial processes. Compared with other commonly used methods, experiments on two real-world datasets of solar power generation prediction and heating furnace temperature prediction demonstrate the effectiveness of the proposed method.
In recent years, the big data research group has been committed to the research and application of artificial intelligence, machine learning and other directions in the industrial field, and has made breakthroughs in industrial process prediction, fault diagnosis, intelligent optimization control, industrial big data platform and other aspects, and has made many breakthroughs in tobacco, automobile enterprises Industries such as metallurgy and mining have been demonstrated and validated.
This study has been published on the IEEE Transactions on Industrial Informatics.