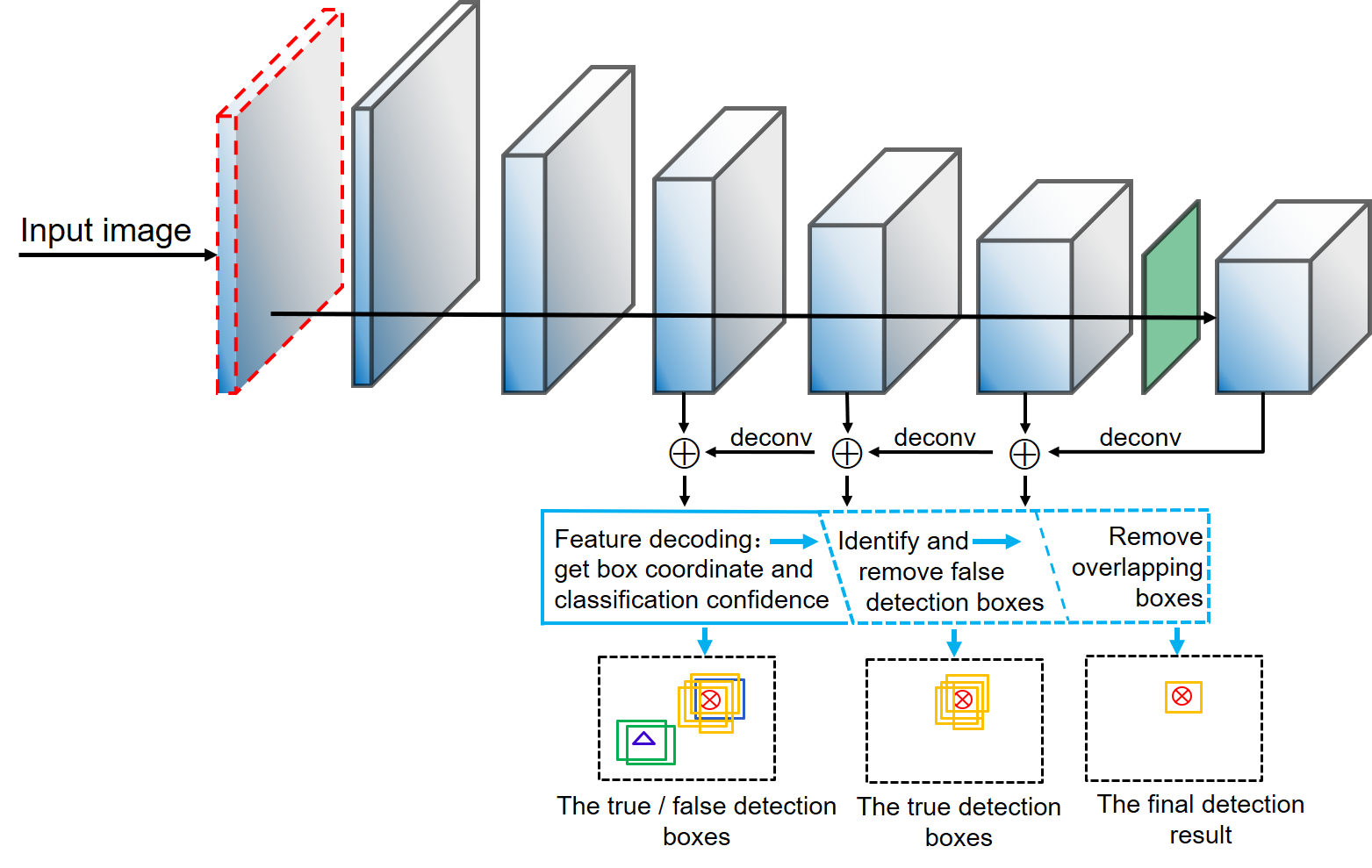
Schematic diagram of detection network structure. (Image by SIACAS)
Object detection methods based on deep learning have been applied in aerial image target detection, but due to the inherent characteristics of aerial images, the results of existing detection methods are unsatisfactory, and there are many cases of false and missed detection.
Recently, a research team at the Shenyang Institute of Automation of Chinese Academy of Sciences (SIACAS) creatively put forward a method to remove the false detection boxes in the aerial image detection results, and designed the corresponding detection network according to the characteristics of the aerial image. The study was published in Sensors.
At present, there are mainly two types of optimization methods in deep-learning-based object detection methods: optimizing the detection network framework for the characteristics of the object to be detected; and optimizing the post-processing methods of detection results. Most of the existing detection algorithms focus on improving the detection ability of the detection network.
In this study, researchers proposed a dual-NMS algorithm was from the perspective of removing the false detection boxes in the detection results. By counting the density of the detection boxes generated around each detected object, the classification confidence and the maximum classification confidence of the corresponding detection box, the false detection boxes in the detection results can be removed autonomously and the accuracy of the detection results can be greatly improved.
Then, researchers take the judgment result of false detection object as the constraint item when training the network, which fundamentally strengthens the ability of the detection network to extract the abstract features of the target to be detected. Therefore, the detection network can automatically avoid the appearance of false detection targets in the process of training.
In addition, the correlation between the same category of targets is introduced into the detection network to enhance the detection ability of the algorithm. Through the correlation between the same categories of objects, the same category of objects is fused into one, which can reduce the difficulty of small object detection in aerial images.
Experimental results show that the dual-NMS can remove nearly 50% of false detection boxes, and the optimized detection network has better performance than the existing algorithm.