Recently, state-of-the-art multitask multiview (MTMV) learning tackles a scenario where multiple tasks are related to each other via multiple shared feature views, which has been used into many fields, e.g., machine learning and computer vision. However, in many real-world scenarios where a sequence of the multiview task comes, retraining previous tasks with MTMV models request higher storage and computational cost.
To address this challenge, the Machine Intelligence research group at Shenyang Institute of automation (SIA), the Chinese Academy of Sciences (CAS) proposes a continual multi-view task learning model that integrates deep matrix factorization and sparse subspace learning in a unified framework, which is termed deep continual multi-view task learning (DCMvTL).
The study was published in IEEE TRANSACTIONS ON NEURAL NETWORKS AND LEARNING SYSTEMS titledContinual Multiview Task Learning via Deep Matrix Factorization.
When a new multi-view task arrives, DCMvTL first adopts a deep matrix factorization technique to capture hidden and hierarchical representations for this new coming multi-view task while accumulating the fresh multi-view knowledge in a layerwise manner.
Then, a sparse subspace learning model is employed for the extracted factors at each layer and further reveals cross-view correlations via a self-expressive constraint.
Extensive experiments on benchmark datasets demonstrate the effectiveness of the proposed DCMvTL model compared with the existing state-of-the-art MTMV and lifelong multi-view task learning models.
This study was supported by National Natural Science Foundation of China and the State Key Laboratory of Robotics.
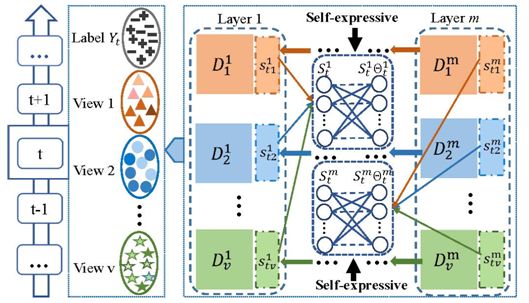
Demonstration of our DCMvTL framework, where different colors correspond to different views.(Photo provided by SUN Gan)
Contact:
SUN Gan
sungan@sia.cn