Recently, Shenyang Automation Research Institute of Chinese Academy of Sciences has made important progress in the field of smart grid data analysis, and proposed a single classification support vector machine anomaly data detection model for smart grid data analysis.
Under the leadership of professors WANG Zhongfeng and SONG Chunhe, the smart grid research group of Shenyang Institute of automation proposed an anomaly detection model suitable for unbalanced data distribution, aiming at the problem that the imbalance of abnormal data and normal data distribution results in significant reduction of anomaly detection accuracy in the process of smart grid data analysis. First the characteristics of smart grid data distribution is analyzed in detail, and the impact of the imbalance of positive and negative samples on the accuracy of data anomaly detection is studied; second, a single classification support vector machine model for smart grid data analysis is proposed; third, due to the difficulty of parameters setting, a novel particle swarm optimization algorithm is proposed, and adaptive speed weighting and adaptive population splitting are introduced, which can improve the convergence speed of the algorithm and help the algorithm jump out of the local optimum. Finally, experiments on the standard benchmark and the actual power system experimental data set prove the effectiveness of the algorithm.
In recent years, the smart grid group of Shenyang Automation Institute has made important progress in network construction, hardware research and development, data collection, analysis, model construction and other fields. It has undertaken a series of important projects in relevant fields, such as national key research and development plan, national grid science and technology project. Meanwhile, the strategic agreements have been signed with State Grid Liaoning Electric Power Co., Ltd., Liaoning Electric Power Research Institute, and other units. Relative worlds have effectively promoted the construction of smart grid.
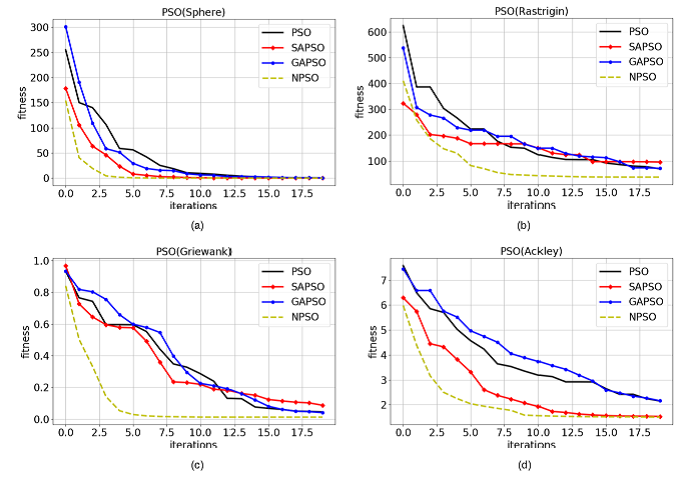
Comparison of the proposed algorithm on different datasets