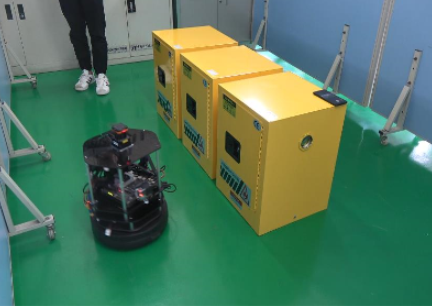
Reseearchers conducting experiement on mobile robot. (Photo by Yanming HU)
Human beings show amazing adaptability when dealing with complex tasks in daily life. This adaptability is the direct embodiment of individual learning ability, which enables human beings to improve their own behavior ability independently and incrementally.
Therefore, if robots can have this ability, it can automatically generate new behavior patterns according to the real-time acquisition of data and cases. This ability shows obvious intelligence, which is called behavioral intelligence.
Recently, researcher at Shenyang Institute of automation, Chinese Academy of Sciences developed a new method developed a new method to improve the behavioral intelligence of robots, related results were published on IEEE Transactions on cognitive and developmental systems.
The research team proposed a new framework of incremental learning method based on Q-Learning and adaptive kernel linear (AKL) model. The framework allows robot to learn new behaviors without forgetting the previous ones. Under the new method, robot behaviors can be evaluated by means of autonomous learning and imitation learning, and the model structure and parameters can be changed in real time using a novel L2-norm kernel recursive least squares (L2-KRLS) algorithm.
The researchers conduct two experiments to validate the performance of the new method. Results show that the proposed framework can incrementally learn behaviors in varying environments. Local -greedy policy-based Q-learning is faster than existing Q-learning algorithms. At present, this achievement has been applied in robot autonomous navigation.
This research is supported by National Nature Science Foundation of China and State Key Laboratory of robotics.