|
User attribute discovery with missing labels |
Author: |
|
Update times: |
2017-12-01 |
|
| Print | Close | Text Size: A A A |
|
|
In this paper, we focus on user attribute analysis by recasting such a problem as a multi-task learning issue, where each attribute is considered as an independent task. In comparison with traditional data analysis, the missing labels problem broadly presents for smart sensor data due to some objective / subjective factors, where the label incompleteness increases the difficulty significantly. Therefore, we design a semi-supervised multi-task learning model (S2MTL) to handle the missing labels issue. For modeling, we integrate the matrix factorization to learn the mapping feature dictionary and attribute space information simultaneously, and adopt the pairwise affinity similarity to incorporate the unlabeled data information, where the low rank property and model efficiency can be well controlled. For model optimization, we convert our model as two individual convex subproblems with one non-smooth, and implement an alternating direction method to generate an efficient optimal solution. State-of-the-art models have validated the effectiveness and efficiency of our proposed model via extensive experiments and comparisons, on two public datasets and our new smart building dataset. 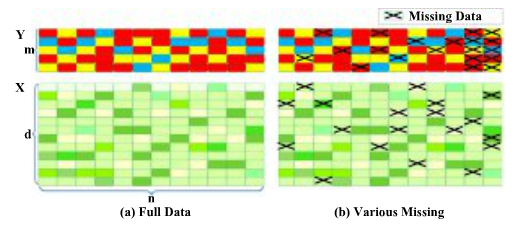
multi-task learning with (a) fully labeled of both X and Y; (b) partially labeled data with various missing entries, such as missing data X, partially missing labels yi and completely missing labels yi (two columns on the right of Y) (image from the paper) This paper is published on Pattern Recognition,2018,73:33-46.titled User attribute discovery with missing labels. |
|
|