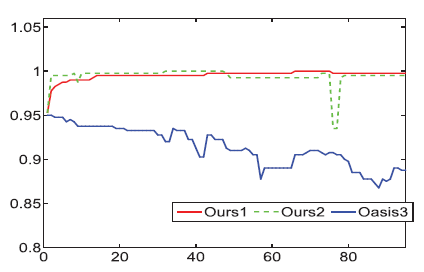
An example of the simulation result, where the x-axis is the number of iterations (10 k per step) and the y-axis is the accuracy. (Image provided by CONG Yang et.al)
Machine learning, as a core technology, has been widely used in various fields, such as computer vision, robot, social network, data digging and big data processing. Traditional machine learning method, based on batch processing mode, requires large quantity of training samples during initialization, and the model cannot update real-time. These two problems have limited the development and application of online learning system.
According to the low rank property of the real data, Cong Yang, researcher from Shenyang Institute of Automation (SIA), the Chinese Academy of Sciences (CAS) proposed a convex online metric learning model, which can lower the complexity of model and solve the overfitting issue. which is commonly seen in online learning model and algorithm. Meanwhile, by modeling the statistics expectation as the object function, the property of model convergence can be justified in theory as well.
Papers related to this result were published on IEEE Transactions on Circuits and Systems for Video Technology (Yang Cong, et al, Speeded up Low Rank Online Metric Learning for Object Tracking, IEEE Transactions on Circuits and Systems for Video Technology, 2014 DOI: 10.1109/TCSVT.2014.2355692), Transactions on Image Processing (Yang Cong, Ji Liu, Junsong Yuan, Jiebo Luo, Self-supervised Online Metric Learning with Low Rank Constraint for Scene Categorization, IEEE Transactions on Image Processing, v22, n 8, pp 3179 - 3191, 2013)和Springer (Yang Cong, Ji Liu, Junsong Yuan, Jiebo Luo, Low-Rank Online Metric Learning, Page 203-233, in Yun Fu, Low-Rank and Sparse Modeling for Visual Analysis, Springer, 2014 ISBN: 978-3-319-11999-1 (Print) 978-3-319-12000-3 (Online).
This research was supported by Youth Innovation Promotion Association, CAS, State Key Laboratory of Robotics, National Natural Science Foundation, and the National High Technology Research and Development Program("863"Program). Some findings have been successfully applied in real projects.
The researcher has been devoted to research on online learning theory and global anomaly recognition, and has achieved a series of original theory and application results. Papers have been published on IEEE CVPR(2009,2011),IEEE Trans on Multimedia(2012),IEEE Trans. on Image Processing (2013), Pattern Recognition (2013,2014), IEEE Trans. on Circuits and Systems for Video Technology (2014)
Contact:
CONG Yang
Email: congyang81@gmail.com
Shenyang Institute of Automation