For its unique technology superiority, endoscopy has been widely used in medical diagnosis, for example, in gastroscopy. In recent years, some computer aided diagnosis algorithms have been proposed in order to assist medical experts in reducing their manual work and improving the accuracy of medical diagnosis. However, for endoscopy image analysis, since no single feature can represent the image properly, multiple features are heuristically combined into one high-dimensional feature vector to complement each other. And one other problem is, it is difficult to select the most discriminating features, and some useless features are also inevitably combined, which result in lower accuracy and increase complexity.
In order to solve the problem, researchers from Shenyang Institute of Automation (SIA), Chinese Academy of Sciences, design a new feature selection model via group sparsity, Deep Sparse Support Vector Machine (DSSVM) which can select both feature units and feature dimensions simultaneously and avoids extracting useless feature units. The difference between our algorithm and most traditional feature selection models is shown in Figure 1.
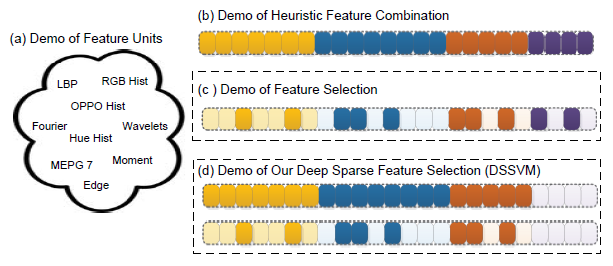
Figure 1: The demonstration: (a) The pool of original individual feature units, i.e., single feature; (b) The heuristic feature combination, e.g., four kinds of feature units denoted by different colors are combined to complement each other and each feature unit contains several feature dimensions demonstrated by cubic; (c) Assigning a weight to each dimension by most of the traditional feature selection models, i.e., the deeper the color of the cubic is, the more important the corresponding feature dimension will be; (d) Selecting useful feature units and feature dimensions inside concurrently selected feature units by our Deep Sparse SVM (DSSVM) model. (Image provided by WANG Shuai et.al)
The computer aided endoscopy diagnosis framework is for warning / supporting and does not make a final decision / diagnosing and replace human experts, and it just detects various abnormalities in esophagus and stomach rather than classifying lesions, such as cancer, bleeding, polyps and ulcers. To achieve this, two key technologies are utilized, feature representation and diagnostic / classification modeling. For feature representation, the researchers first segment an image into superpixels, which is more flexible and accurate than patch-based methods. Then they extract various color and texture features and combine them into one feature vector to represent each superpixel, in which the color and texture features are complementary to each other.
For diagnostic / classification modeling, due to the impact of uneven illumination, such as light saturation by reflection and shadowing, the image quality of some regions in endoscopy image is so poor that even human experts are not able to make a correct decision. Therefore, before fed into our DSSVM model, the researchers first assess the image quality, and then for the superpixel with acceptable image quality, they classify whether it is normal or abnormal. All these are based on our DSSVM model. Finally, a spatial smoother is adopted to improve the robustness of the algorithm.
The ROC curves by comparing our DSSVM with the state-of-the-art methods are shown in Figure 2 using both I800 sub-dataset and I3000 sub-dataset. For each dataset, the performance of the proposed model DSSVM is better than the other three methods.
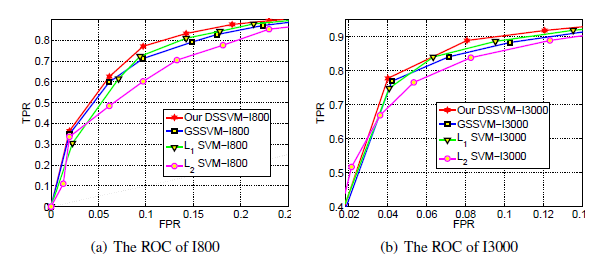
Figure 2: The ROC curves by comparing our DSSVM with the state-of-the-art methods (GSSVM, L1SVM and L2SVM) using (a) I800 sub-dataset with pixel-level groundtruth and (b) I3000 subdatasets with frame-level groundtruth. (Image provided by WANG Shuai et.al)
This work is in press on the journal of Pattern Recognition. It was supported by National Science and Technology Support Program (2012BAI14B03), NSFC (61105013, 61375014) and also the foundation of Chinese Scholarship Council.
CONTACT:
Shuai Wang
Dr.Yang Cong, Associate Professor
Shenyang Institute of Automation, Chinese Academy of Sciences
Email: shuaiwang@sia.cn;congyang@sia.cn