Nowadays, prosthetic devices have helped many disabled people who have lost limbs to deal with their daily life. Advanced commercial prosthetic hand such as Otto Bock hand, i-Limb hand, and Smart hand have been able to achieve some motions like human hands. The approach using electromyography (EMG) signals from amputee persons’ remnant muscles to control prosthetic devices is beneficial to restore the missing functionality of amputated limbs, and it has a history of about 40 years.
In an EMG pattern recognition system, feature extraction is the process that converts original sEMG signals to a compact and informative set of features. Traditional methods such as time-domain and frequency-domain analyzing methods have been widely used in EMG pattern recognition and they have a good capability to track muscular changes. However, these methods are not effective in detecting the critical feature of sEMG signals during transient human movements. In order to recognize more motion patterns, more channels of sEMG singles and more kinds of features are used to increase the information extracted from sEMG signals.
Recently, rearchers from Shenyang Institute of Automation have conducted a study on EMG pattern recognition based on principal component analysis (PCA) and linear discriminant analysis (LDA) for an anthropomorphic robotic hand. In this study, the pattern recognition system consists of the feature extraction including five kinds of analysis methods, PCA feature projection, LDA feature projection and minimum distance classifier (MDC), as shown in Fig. 1. Nine kinds of hand/wrist motions were selected, and four channels of sEMG signals from human’s forearm were collected.
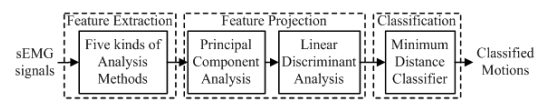
Fig. 1. Block diagram of EMG pattern recognition system. (Image provided by Daohui ZHANG et.al)
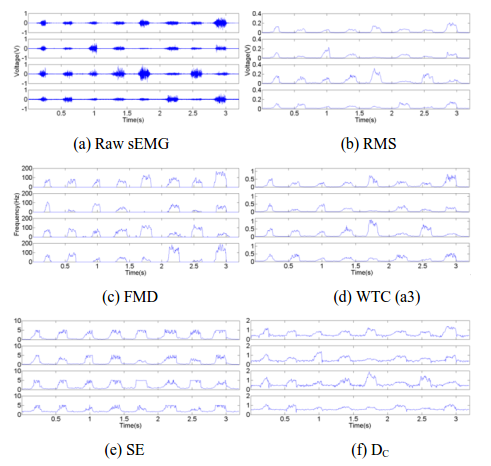
Fig.2. Some features extracted from 4 channels of sEMG signals. (Image provided by Daohui ZHANG et.al)
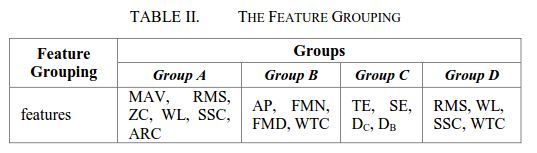
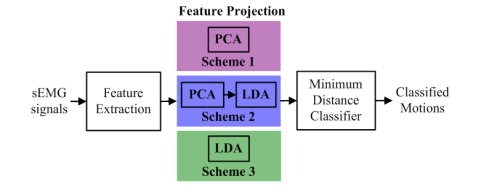
Fig. 3. Schematic diagram of the three feature projection schemes. (Image provided by Daohui ZHANG et.al)
Experimental results indicated that PCA+LDA projection didn’t improve the recognition performance much compared to LDA projection, and the time-domain features and wavelet transform features were able to obtain a better performance on both the classification accuracy and the processing time compared to the frequency-domain features, nonlinear entropy features and fractal analysis features.
This work was presented at the 2014 IEEE International Conference on Robotics & Automation (ICRA) which was held in Hong Kong during May 31 to June 7, 2014.
Contact:
ZHANG Daohui
zhangdaohui@sia.cn
Shenyang Institute of Automation