Anomaly detection, also named as outlier detection, is applicable in a variety of applications, such as intrusion detection, fraud detection, fault detection, system health monitoring, event detection in sensor networks, and detecting eco-system disturbances. The Oxford English Dictionary defines abnormal as:
deviating from the ordinary type, especially in a way that is undesirable or prejudicial; contrary to the normal rule or system; unusual, irregular, aberrant.
According to the definition, the abnormal events can be identified as irregular events from normal ones. Thus, the task is to identify abnormal (negative) events given the normal (positive) training samples.
To address this problem, most conventional algorithms intend to detect testing sample with lower probability as anomaly by fitting a probability model over the training data. As a high-dimensional feature is essential to better represent the event and the required number of training data increases exponentially with the feature dimension, it is unrealistic to collect enough data for density estimation in practice. For example, for our global abnormal detection, there are only 400 training samples with dimension of 320. With such a limited training samples, it is difficult to even fit a Gaussian model. Thus, the state-of-the-art abnormal event detection methods cannot handle this headache issue well.
To handle this issue, the researchers from Shenyang Institute of Automation, the Chinese Academy of Sciences (CAS) propose a new abnormal measurement criteria, sparse reconstruction cost (SRC) based on the L1 minimization over the normal dictionary to measure the normalness of the testing sample, as

The demonstration is shown in Fig.1. By introducing the prior weight of each basis during sparse reconstruction, the proposed SRC is more robust compared to other outlier detection criteria. To condense the over-completed normal basis into a compact dictionary, a novel dictionary selection method with group sparsity constraint is designed and can be solved by the standard convex optimization algorithm. By using different types of basis, the researchers provide a unified solution to detect both local and global abnormal events in crowded scene, which can also be extended to online event detection by an incremental self-update mechanism. Experiments on benchmark datasets for both global and local abnormal events and the comparison to the state-of-the-art methods are shown in Fig.2 and Fig.3.
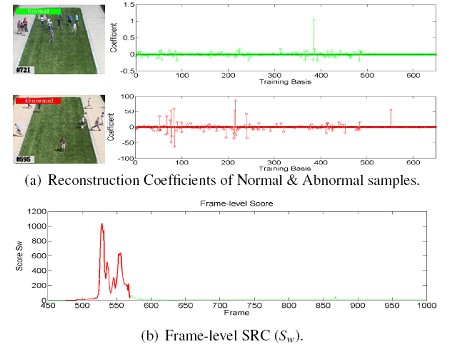
Fig 1 (a) Top left: the normal sample; top right: the sparse reconstruction coefficients; bottom left: the abnormal sample; bottom right: the dense reconstruction coefficients. (b) Frame-level Sparsity Reconstruction Cost (SRC): the red/green color corresponds to abnormal/normal frame, respectively. It shows that the Sw of abnormal frame is greater than normal ones, and we can identify abnormal events accordingly. (Image by Prof. CONG Yang et al.)

Fig 2 The qualitative results of the global abnormal event detection for three sample videos from UMN dataset. The top row represents snapshots of the result for a video in the dataset. At the bottom, the ground truth bar and the detection result bar show the labels of each frame for that video, where green color denotes the normal frames and red corresponds to abnormal frames.(Image by Prof. CONG Yang et al.)
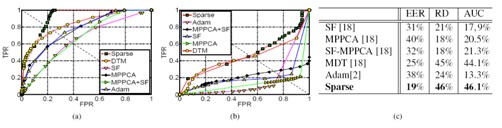
Fig 3 (a) Frame-level ROCs for Ped1 Dataset, (b) Pixel-level ROCs for Ped1 Dataset, (c) Quantitative comparison of our method with [18][2]: EER is equal error rate; RD is rate of detection; and AUC is the area under ROC. (Image by Prof. CONG Yang et al.)
This work was published on the journal of Pattern Recognition, Volume 46, Issue 7, July 2013, 1851-1864.
It was partly supported by the Natural Science Foundation of China (61105013), the National Science and Technology Pillar Program (2012BAI14B03).
CONTACT:
Prof. CONG Yang
Shenyang Institute of Automation, the Chinese Academy of Sciences
Email: congyang81@gmail.com