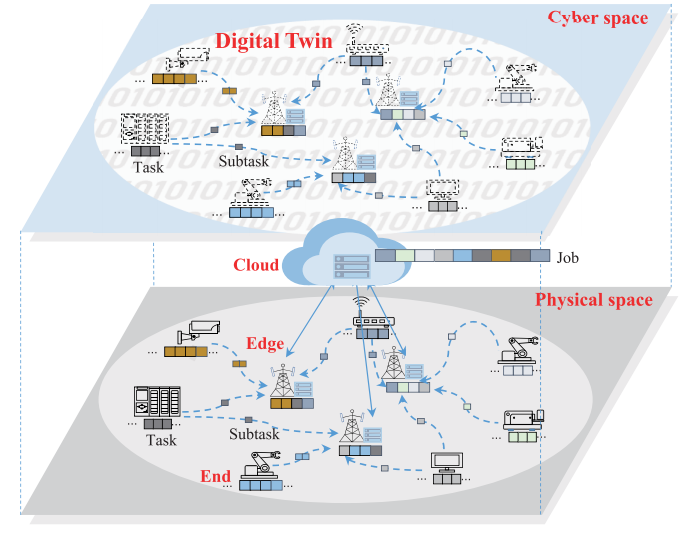
The digital twin model of wireless networks
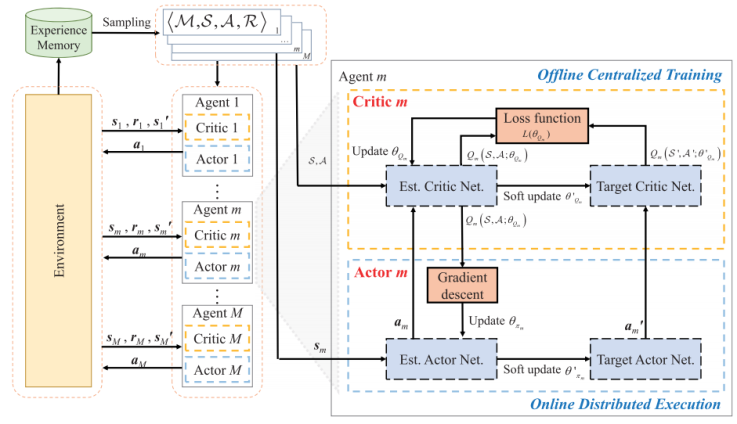
End-edge collaborative scheduling based on multi-agent deep reinforcement learning
Edge computing technology, which can provide enough computing resources for end devices and effectively reduce tasks’ transmission and processing delays by deploying computing resources nearby end devices, is regarded as a key technology for enabling high real-time and high reliable applications such as industrial control. However, it is extremely easy to cause the competition of computation resource and the congestion of wireless network during task offloading due to the heterogeneous tasks and scarcity of communication resources.
To solve this problem, the industrial 5G team of Shenyang Institute of Automation, Chinese Academy of Sciences (SIACAS), proposed a digital twin driven end-edge collaborative scheduling method for heterogeneous tasks and resources. The achievement was published on the academic journal IEEE Journal on Selected Areas in Communications titled Digital Twin Driven Collaborative Scheduling for Heterogeneous Tasks and Edge End Resource via Multi Agent Deep Reinforcement Learning.
In this study, the research team used digital twin technology to virtualize heterogeneous tasks such as sensing/control, heterogeneous computing resources such as CPU/GPU, and heterogeneous communication resources such as channel/power.
Then, by fully considering the deadline requirements of heterogeneous tasks, the computation types and processing capabilities of end-edge devices, the resource estimation deviation of digital twins, the maximum transmit power and the tolerable peak interference powers of end devices, they established a job completion time minimization problem and proposed the end-edge collaborative scheduling method based on multi-agent deep reinforcement learning.
This method synchronously completes computation type matching, end-edge task division, computation resource allocation, and power control by offline centralized training and online distributed execution. In this way, the method achieves end-edge collaborative scheduling of heterogeneous tasks and communication-computation resources, meeting the diverse requirements of heterogeneous tasks.
In recent years, the research team have focused on the integration and innovation of 5G and industrial manufacturing technology, and have made a series of research progress in protocol design, optimization and scheduling, standard formulation, system verification, and other aspects of industrial 5G networks. The results have been published in academic journals such as IEEE Transactions on Industrial Informatics, IEEE Communications Magazine, IEEE Network, and Engineering.